Metrics of matching (advanced)¶
Example of the more functions to plot metrics of matching, using non-standard quantities of the catalogs
Table of Contents
1 Generate random data and add to catalog
2 Match catalogs
3 Recovery rate
3.1 Binned plot
3.2 Sky plots
4 Distances of matching
5 Scaling Relations
%load_ext autoreload
%autoreload 2
import numpy as np
import pylab as plt
Generate random data and add to catalog¶
# For reproducibility
np.random.seed(1)
from support import gen_cluster
input1, input2 = gen_cluster(ra_min=0, ra_max=30, dec_min=0, dec_max=30)
Initial number of clusters (logM>12.48): 2,740
Clusters in catalog1: 835
Clusters in catalog2: 928
from clevar import ClCatalog
c1 = ClCatalog('Cat1', ra=input1['RA'], dec=input1['DEC'], z=input1['Z'], mass=input1['MASS'],
mass_err=input1['MASS_ERR'], z_err=input1['Z_ERR'])
c2 = ClCatalog('Cat2', ra=input2['RA'], dec=input2['DEC'], z=input2['Z'], mass=input2['MASS'],
mass_err=input2['MASS_ERR'], z_err=input2['Z_ERR'])
# Format for nice display
for c in ('ra', 'dec', 'z', 'z_err'):
c1[c].info.format = '.2f'
c2[c].info.format = '.2f'
for c in ('mass', 'mass_err'):
c1[c].info.format = '.2e'
c2[c].info.format = '.2e'
/home/aguena/.local/lib/python3.9/site-packages/clevar-0.13.2-py3.9.egg/clevar/catalog.py:267: UserWarning: id column missing, additional one is being created.
warnings.warn(
Match catalogs¶
from clevar.match import ProximityMatch
from clevar.cosmology import AstroPyCosmology
match_config = {
'type': 'cross', # options are cross, cat1, cat2
'which_radius': 'max', # Case of radius to be used, can be: cat1, cat2, min, max
'preference': 'angular_proximity', # options are more_massive, angular_proximity or redshift_proximity
'catalog1': {'delta_z':.2,
'match_radius': '1 mpc'
},
'catalog2': {'delta_z':.2,
'match_radius': '10 arcsec'
}
}
cosmo = AstroPyCosmology()
mt = ProximityMatch()
mt.match_from_config(c1, c2, match_config, cosmo=cosmo)
## ClCatalog 1
## Prep mt_cols
* zmin|zmax from config value
* ang radius from set scale
## ClCatalog 2
## Prep mt_cols
* zmin|zmax from config value
* ang radius from set scale
## Multiple match (catalog 1)
Finding candidates (Cat1)
* 719/835 objects matched.
## Multiple match (catalog 2)
Finding candidates (Cat2)
* 721/928 objects matched.
## Finding unique matches of catalog 1
Unique Matches (Cat1)
* 719/835 objects matched.
## Finding unique matches of catalog 2
Unique Matches (Cat2)
* 720/928 objects matched.
Cross Matches (Cat1)
* 719/835 objects matched.
Cross Matches (Cat2)
* 719/928 objects matched.
Recovery rate¶
Compute recovery rates, in the main functions they are computed in mass and redshift bins. Here a more advanced use where different quantities can be used. There are several ways they can be displayed: - Single panel with multiple lines - Multiple panels - 2D color map
To use this, import the ClCatalogFuncs
package from recovery
. It
contains the functions: - plot
- plot_panel
- plot2D
There functions have the names of the columns as arguments, so you can use different columns available in the catalogs.
from clevar.match_metrics.recovery import ClCatalogFuncs as r_cf
zbins = np.linspace(0, 2, 11)
mbins = np.logspace(13, 14, 5)
Binned plot¶
The recovery rates are shown as a function of col1
in col2
bins.
They can be displayed as a continuous line or with steps:
info = r_cf.plot(c1, col1='z', col2='mass', bins1=zbins, bins2=mbins,
matching_type='cross', legend_format=lambda x: f'10^{{{np.log10(x)}}}')
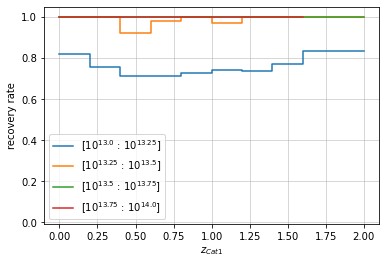
They can also be transposed to be shown as a function of mass in redshift bins.
info = r_cf.plot_panel(c1, col1='z', col2='mass', bins1=zbins, bins2=mbins,
matching_type='cross', label_format=lambda x: f'10^{{{np.log10(x)}}}')
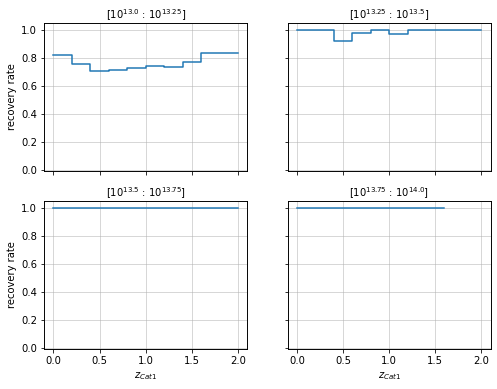
info = r_cf.plot2D(c1, col1='z', col2='mass', bins1=zbins, bins2=mbins,
matching_type='cross', scale2='log')
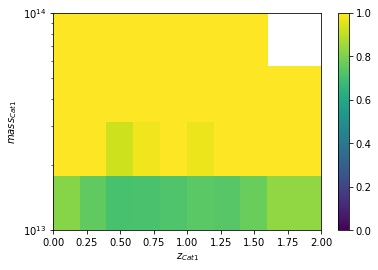
Sky plots¶
It is possible to plot the recovery rate by positions in the sky. It is done based on healpix pixelizations:
info = r_cf.skyplot(c1, matching_type='cross', nside=16, ra_lim=[-10, 50], dec_lim=[-5, 35])
/home/aguena/miniconda3/envs/clmmenv/lib/python3.9/site-packages/healpy/projaxes.py:920: MatplotlibDeprecationWarning: You are modifying the state of a globally registered colormap. In future versions, you will not be able to modify a registered colormap in-place. To remove this warning, you can make a copy of the colormap first. cmap = copy.copy(mpl.cm.get_cmap("viridis"))
newcm.set_over(newcm(1.0))
/home/aguena/miniconda3/envs/clmmenv/lib/python3.9/site-packages/healpy/projaxes.py:921: MatplotlibDeprecationWarning: You are modifying the state of a globally registered colormap. In future versions, you will not be able to modify a registered colormap in-place. To remove this warning, you can make a copy of the colormap first. cmap = copy.copy(mpl.cm.get_cmap("viridis"))
newcm.set_under(bgcolor)
/home/aguena/miniconda3/envs/clmmenv/lib/python3.9/site-packages/healpy/projaxes.py:922: MatplotlibDeprecationWarning: You are modifying the state of a globally registered colormap. In future versions, you will not be able to modify a registered colormap in-place. To remove this warning, you can make a copy of the colormap first. cmap = copy.copy(mpl.cm.get_cmap("viridis"))
newcm.set_bad(badcolor)
/home/aguena/miniconda3/envs/clmmenv/lib/python3.9/site-packages/healpy/projaxes.py:202: MatplotlibDeprecationWarning: Passing parameters norm and vmin/vmax simultaneously is deprecated since 3.3 and will become an error two minor releases later. Please pass vmin/vmax directly to the norm when creating it.
aximg = self.imshow(
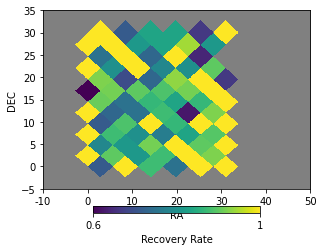
Distances of matching¶
The main functions in distances
can already be binned along other
quantities of the catalog and do not require a more advanced use.
Nonetheless it also has a ClCatalogFuncs
package and can be used
with the same formalism:
from clevar.match_metrics.distances import ClCatalogFuncs as d_cf
info = d_cf.central_position(c1, c2, 'cross', radial_bins=20, radial_bin_units='degrees',
col2='z', bins2=zbins[::4])
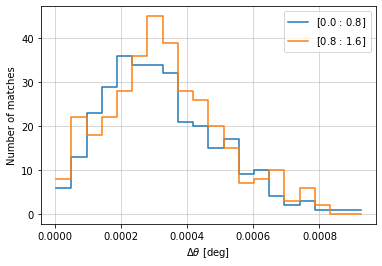
info = d_cf.redshift(c1, c2, 'cross', redshift_bins=20,
col2='z', bins2=zbins[::4], normalize='cat1')
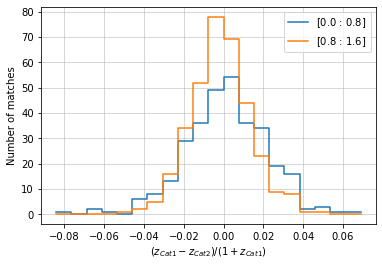
Scaling Relations¶
Here you will be able to evaluate the scaling relations of any two
quantities of the matched catalogs. Import the ClCatalogFuncs
package from scaling
, the functions of this package are: - plot
:
Scaling relation of a quantity - plot_color
: Scaling relation of a
quantity with the colors based on a 2nd quantity - plot_density
:
Scaling relation of a quantity with the colors based on density of
points - plot_panel
: Scaling relation of a quantity divided in
panels based on a 2nd quantity - plot_color_panel
: Scaling relation
of a quantity with the colors based on a 2nd quantity in panels based on
a 3rd quantity - plot_density_panel
: Scaling relation of a quantity
with the colors based on density of points in panels based on a 2rd
quantity - plot_metrics
: Metrics of quantity scaling relation. -
plot_density_metrics
: Scaling relation of a quantity with the colors
based on density of points with scatter and bias panels - plot_dist
:
Distribution of a quantity, binned by other component in panels, and an
optional secondary component in lines. - plot_dist_self
:
Distribution of a quantity, binned by the same quantity in panels, with
option for a second quantity in lines. Is is useful to compare with
plot_dist results.
take the name of the quantity to be binned:
from clevar.match_metrics.scaling import ClCatalogFuncs as s_cf
info = s_cf.plot(c1, c2, 'cross', col='mass', xscale='log', yscale='log')
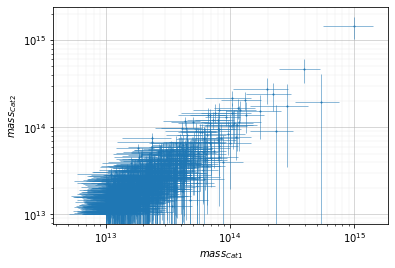
info = s_cf.plot(c1, c2, 'cross', col='mass', xscale='log', yscale='log',
col_color='z', add_err=False)
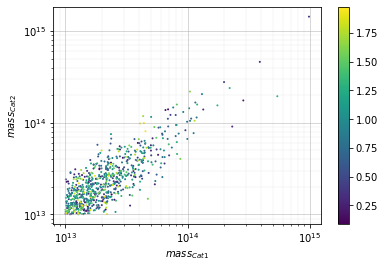
info = s_cf.plot_density(
c1, c2, 'cross', col='mass',
xscale='log', yscale='log', add_err=False,
add_fit=True, fit_bins1=5, fit_log=True)
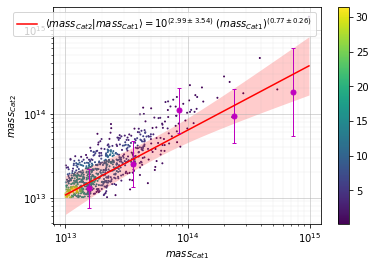
info = s_cf.plot_panel(
c1, c2, 'cross', col='mass', xscale='log', yscale='log',
col_panel='z', bins_panel=zbins[::2])
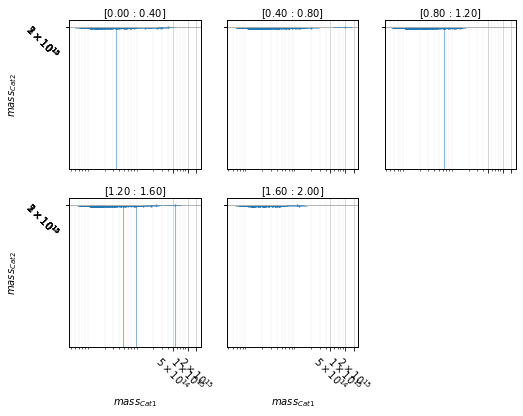
info = s_cf.plot_panel(
c1, c2, 'cross', col='mass', xscale='log', yscale='log',
col_panel='z', bins_panel=zbins[::2],
col_color='z')
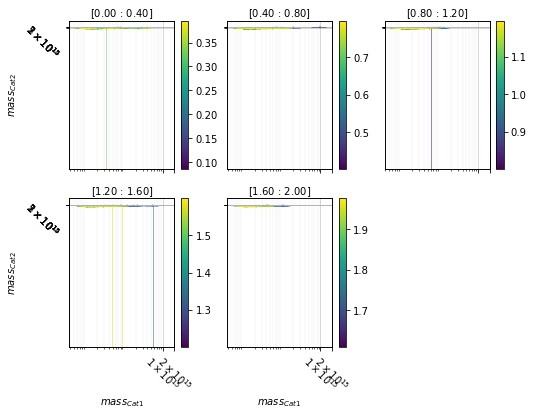
info = s_cf.plot_density_panel(
c1, c2, 'cross', col='mass', xscale='log', yscale='log',
col_panel='z', bins_panel=zbins[::2])
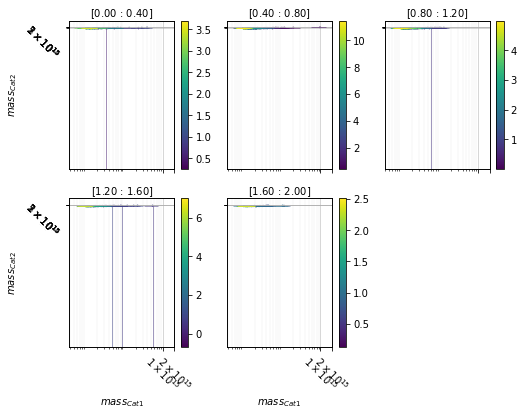
info = s_cf.plot_metrics(c1, c2, 'cross', col='z', mode='diff_z', label1='z1')
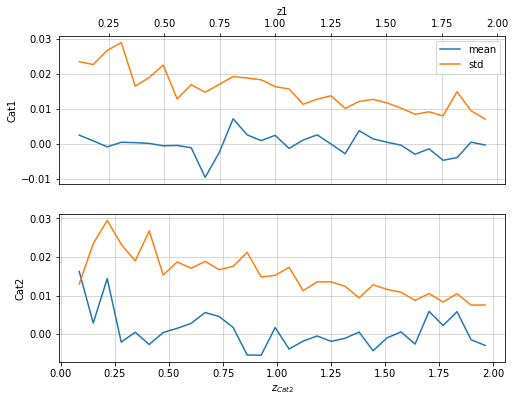
info = s_cf.plot_density_metrics(c1, c2, 'cross', col='z', metrics_mode='diff_z')
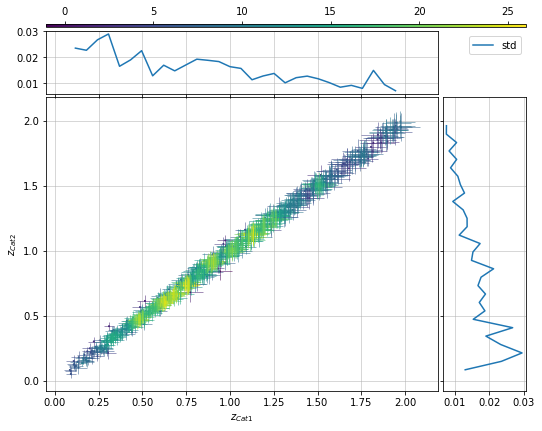
info = s_cf.plot_density_metrics(
c1, c2, 'cross', col='mass', metrics_mode='log',
scale1='log', scale2='log')
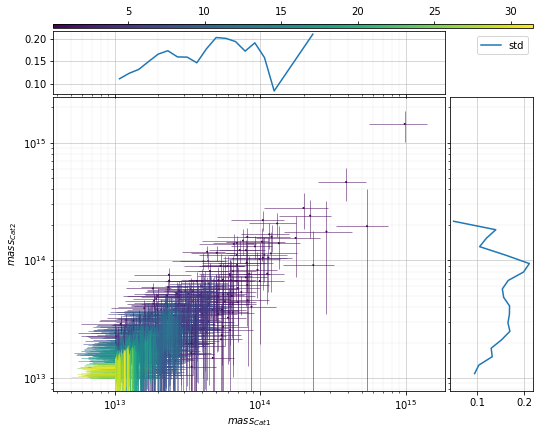
info = s_cf.plot_density_dist(
c1, c2, 'cross', col='mass', metrics_mode='log',
scale1='log', scale2='log', add_err=False)
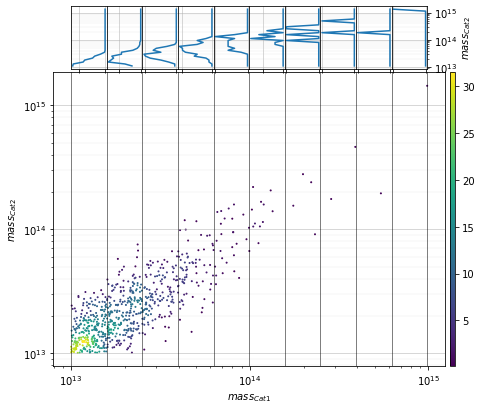
zbins2 = np.linspace(0, 1.2, 7)[1:]
rbins2 = [1, 5.5, 25, 50, c2['mass'].max()]
bins1 = 10**np.histogram(np.log10(c1['mass']), 20)[1]
info = s_cf.plot_dist(
c1, c2, 'cross', 'mass',
bins1=21, bins2=[10**13.0, 10**13.2, 10**13.5, 1e14, 1e15],
col_aux='z', bins_aux=4, shape='line',
log_vals=True, fig_kwargs={'figsize':(15, 6)})
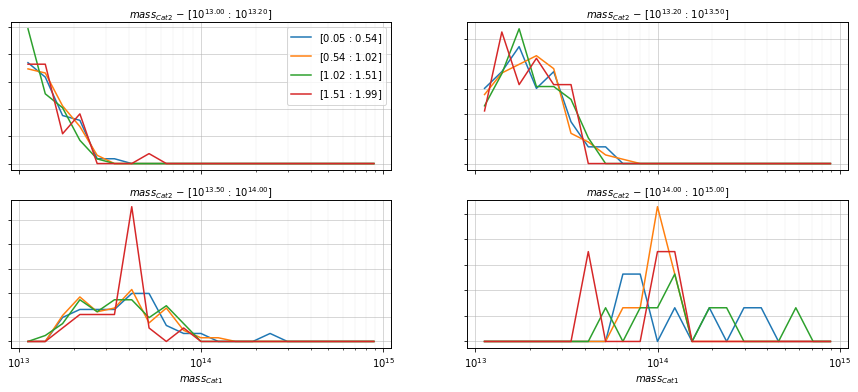